Improved Mutual Mean-Teaching
for Unsupervised Domain Adaptive Re-ID
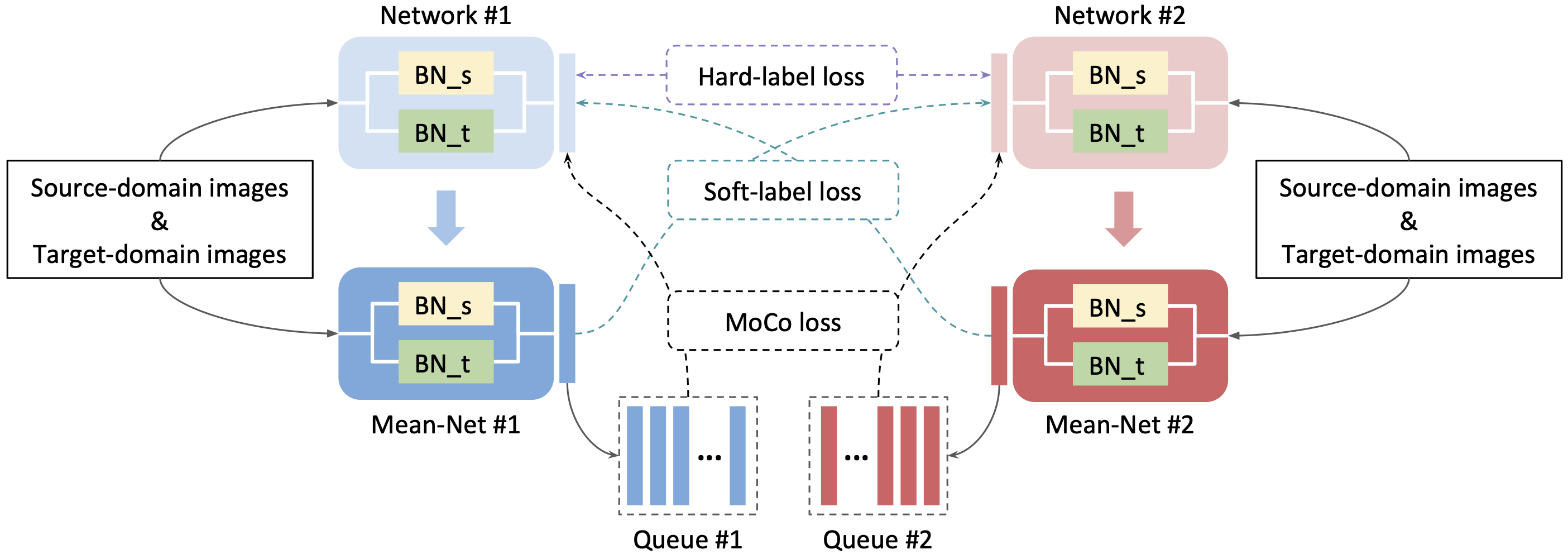
Abstract
In this technical report, we present our submission to the VisDA Challenge in ECCV 2020 and we achieved one of the top-performing results on the leaderboard. Our solution is based on Structured Domain Adaptation (SDA) and Mutual Mean-Teaching (MMT) frameworks. SDA, a domain-translation-based framework, focuses on carefully translating the source-domain images to the target domain. MMT, a pseudo-label-based framework, focuses on conducting pseudo label refinery with robust soft labels. Specifically, there are three main steps in our training pipeline. (i) We adopt SDA to generate source-to-target translated images, and (ii) such images serve as informative training samples to pre-train the network. (iii) The pre-trained network is further fine-tuned by MMT on the target domain. Note that we design an improved MMT (dubbed MMT+) to further mitigate the label noise by modeling inter-sample relations across two domains and maintaining the instance discrimination. Our proposed method achieved 74.78% accuracies in terms of mAP, ranked the 2nd place out of 153 teams.
Materials
Citation
@misc{ge2020improved, title={Improved Mutual Mean-Teaching for Unsupervised Domain Adaptive Re-ID}, author={Yixiao Ge and Shijie Yu and Dapeng Chen}, year={2020}, eprint={2008.10313}, archivePrefix={arXiv}, primaryClass={cs.CV} } @inproceedings{ge2020mutual, title={Mutual Mean-Teaching: Pseudo Label Refinery for Unsupervised Domain Adaptation on Person Re-identification}, author={Yixiao Ge and Dapeng Chen and Hongsheng Li}, booktitle={International Conference on Learning Representations}, year={2020}, url={https://openreview.net/forum?id=rJlnOhVYPS} } @misc{ge2020structured, title={Structured Domain Adaptation with Online Relation Regularization for Unsupervised Person Re-ID}, author={Yixiao Ge and Feng Zhu and Rui Zhao and Hongsheng Li}, year={2020}, eprint={2003.06650}, archivePrefix={arXiv}, primaryClass={cs.CV} }